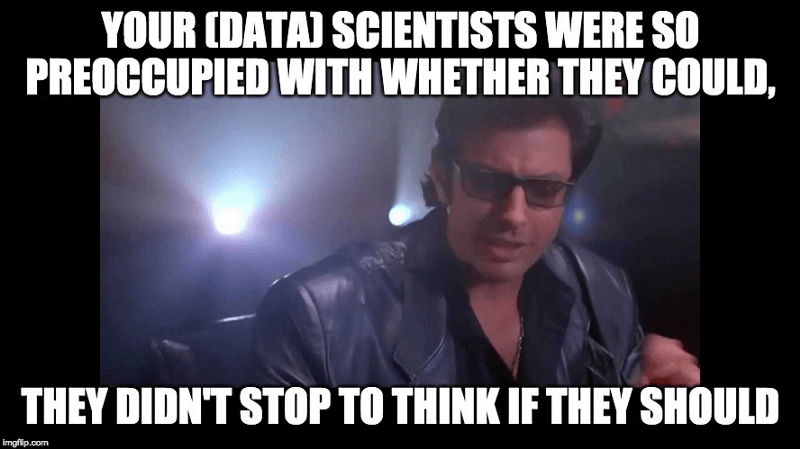
For (and against) partnering for your LTV model
Over the past 10 years of mobile app development, app companies have built marketing tools to support their core businesses. This includes launching ad networks, building ad tracking solutions, creating solutions to combat fraud and probably least controversially, building marketing creative.
As the complexity required to build these tools increases, the success rate decreases. There aren’t many successes to talk of, mainly because the initiative doesn’t fit within their core DNA. Additionally and tragically, it’s not always the case that a company goes through an intentional planning exercise during which the build vs buy vs partner conversation is raised. Often companies start building solutions internally without understanding if it’s the right path to proceed down to execute against the strategic objectives of the business. They “fall into” a business initiative because of a passion project
As the user acquisition industry moves swiftly from optimizing towards CPI to LTV, how to measure LTV (or pLTV — predicted LTV) becomes acutely important. I believe Jeff Goldblum’s quote from Jurassic Park has never been more relevant “Your (data) scientists were so preoccupied with whether or not they could, they didn’t stop to think if they should.”
In this article I’ll outline the reasons I think it might be valuable for a company to build their own LTV models and the case to partner to ensure a company executes in its core business whilst achieving its strategic objectives.
Case for building in-house:
Strategic benefits:
These are hard to quantify without the benefit of hindsight but the case for “owning” an innovation vs partnering is normally the key reason for a company to continue to invest in building vs partnering. Within the realm of LTV modeling — most companies don’t invest enough time into assessing what are the benefits of building an LTV model internally and do they justify the level of investment required to be successful
- Is there a competitive edge for developing your own LTV model?
- Are there viable solutions available in the market to partner with?
- What’s the opportunity cost of investing in this innovation vs other initiatives?
Most companies continue to develop their internal model because they already have a team dedicated to doing this, not because they’ve run through a rigorous planning exercise to understand if this is the right option.
Existing cohort models “work”
Currently, there are 2 ways for marketing / BI teams to build simple models to approximate LTV at the cohort level:
- A multiplier is a method of approximating pLTV using early, actualized data. It’s a number multiplied to the early performance of a cohort of users to extrapolate observed data (normally after 7 days) into the future (up to 1 year). Marketing teams use 7 days worth of data because they need quick feedback loops from user acquisition campaigns to understand how performance is tracking to target. These multipliers are applied at the cohort level, therefore to improve accuracy, a different “multiplier” needs to be applied based on the granularity of the cohort the marketer wishes to track. This can lead to significant levels of complexity in a model once we consider platform, geo and channel
- Multiplying ARPDAU (average revenue per daily active user) by average retention, for a cohort over a specific time period allows another way to approximate pLTV. This methodology doesn’t take into account the cost of the user at the time of acquisition and the same challenges exist with regards to levels of complexity dependent on cohort size level of granularity required.
Both these approaches “work” to the degree a company is prepared to accept the over/underspend into a cohort which may result in a difference of 50% +/- ROAS on their projected marketing spend. This can be seen as the “cost of doing business” versus the alternative of appropriately investing in predicting LTV.
Case for partnering:
Better allocation of DS and Data Eng resources to other tasks
There are a multitude of focus areas a mobile app economy data science team can spend their time on. This article does a great job of highlighting those focus areas specific to gaming companies but there are analogies for non-gaming companies for each of the points noted. Each point essentially falls into two categories:
- Product optimization
- Marketing optimization
It’s the role of the data science team in partnership with the wider org, to understand the highest impact items a DS team can deliver successfully that also deliver strategic value for the company. Working on a “project” basis can deliver quick wins but building a solution to a problem that doesn’t receive ongoing, planned support can mean the initial time and effort investment doesn’t have long term payoffs when looked at through an opportunity cost lens.
It should be clear within the organization that building an LTV model is not a financial planning exercise — it’s a complex data science solution that (unlike financial forecasting) does not have an out of the box, cross-industry accepted solution.
Planning and funding the most strategically beneficial areas for a DS team to work on is most challenging when external factors can change both product and marketing performance. A competitor might launch a similar product, or marketing costs can rise plus a host of other unknowns. It’s critical that whilst planning DS investments, the org is clear about where it can build a strategic, competitive advantage that will continue to receive funding years into the future.
Updating the model:
Where a company has limited DS resources, cohort models can provide an adequate solution. Cohort models don’t account for seasonality; require long time histories and quickly become outdated. Should monetization within the product change or a new channel or geo need to be explored, the model will need to be updated to accommodate this. It’s crucial that the LTV model receives ongoing updates to ensure it remains both directionally useful and ultimately accurate over a reasonable time horizon.
Use cases:
Most DS & Analytics teams build an LTV model almost exclusively for user acquisition purposes but there are many other use cases that can add significant value to the business. We can group these into two main areas:
1. CRM
- Customize user-level push notifications, emails or SMS based on an individual users pLTV and churn probability
2. Product optimization
- Optimize the content for each user based on their pLTV and churn probabilities
- Understand specific churn behavior for high-value cohorts
- Dynamically price items based on their future spend behavior
Having prior data from similar apps, users can ensure an LTV model can be built quickly once a new app is launched.. Although this is an approximation of the LTV, it does have value in providing benchmarks for a new product that can enable marketing decisions to be quickly made against the projections. Using data from eg: 30 other match 3 titles provides a good level of understanding on how a new product might perform on a specific platform, geo, or user acquisition source.
Automation
Building a LTV model can help a user acquisition team better allocate marketing spend however, this should be the first step in executing against a long-term vision improving user acquisition efforts. In fact, it’s likely a better use of resources to invest in automating user acquisition efforts earlier than to continue to invest in improving an LTV model that’s already directionally correct.
In summary, it’s truly a strategic initiative to build and support an LTV model internally with payoffs and costs. The question becomes, “should we partner on this initiative” or, “Is this our strategic advantage”. That’s for every business to answer for themselves.