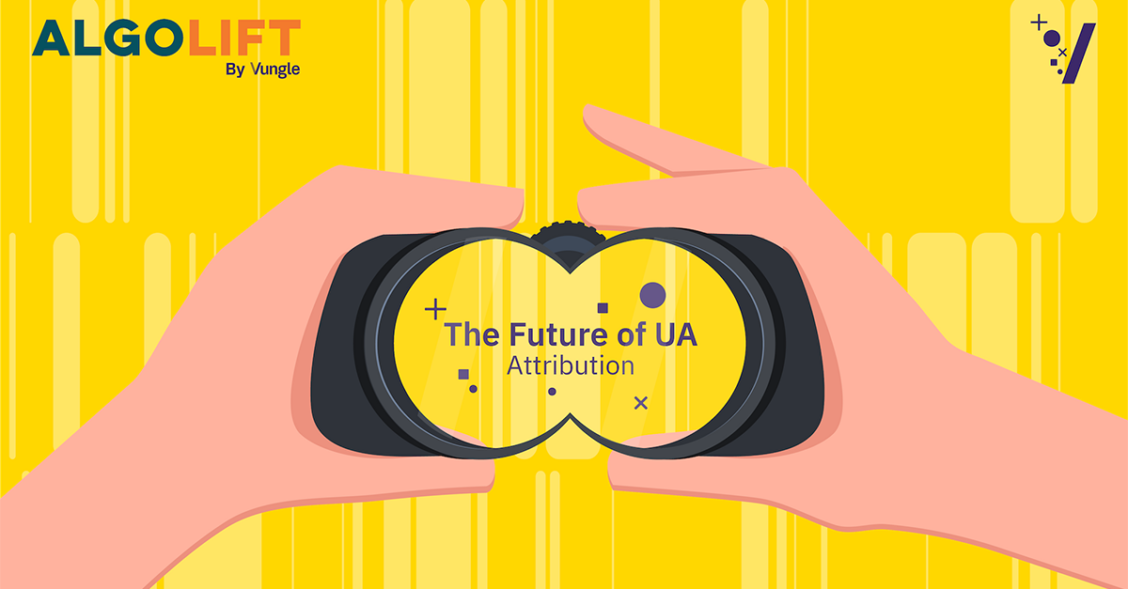
The Future of UA: Attribution and Its Ongoing Disruption
In the “The Future of UA” series for the Vungle blog, we unpack key trends or issues confronting mobile marketers in the ever-evolving mobile industry. Part three in the series: attribution and its ongoing disruption.
With the advent of smartphones, mobile marketers could reach an audience of consumers who carried around with them the most personal piece of technology in history.
These smartphone devices track every swipe, finished level, completed meditation, and in-app purchase—all trackable down to the user level.
Before the smartphone age, no digital marketer had this level of knowledge at their fingertips about their target audience. The personal nature of the mobile device differentiated it from digital marketing for desktop web. In comparison to each person in a household setting having their own smartphone, a personal computer in the same setting was generally used by multiple people. And mobile marketing was a world away from offline marketing, where the objective was to tie disparate offline marketing data back to real-world product purchases.
Core to marketing, and specifically marketing on mobile devices, is the process of attribution, which can be defined as the attempt to:
“Give the right amount of credit to a campaign or channel/ad network for driving downstream marketing metrics such as site visits, installs, or purchases.”
A crucial piece of data that differentiated marketing on mobile from other digital platforms was the ability to identify individual devices (without knowing personal information about the device owner). This was achieved by various device IDs provided by Apple and Google that changed over time as each tech giant evolved their view on user privacy. Initially, mobile marketers had access to UDID (Unique Device ID) on iOS and Android ID on Android, both of which were persistent IDs that couldn’t be user reset. Later, UDID and Android ID were replaced with IDFA (Identifier for Advertisers) on iOS and GAID (Google Ad ID) on Android, both of which were resettable IDs that allowed for users to opt out of tracking for marketing purposes.
Mobile device IDs are the backbone of attribution, ensuring that top-of-the-funnel activity such as impressions and clicks can be matched with downstream metrics such as installs and purchases on a one-to-one basis. By matching such data through mobile device IDs, this means it’s possible to map a user journey from impression to purchase across campaigns and ad networks. For example, a user might view an ad for an app on Facebook mobile, but then search for the product on Google mobile search and click there to install the app and then track the ongoing in-app behavior. Mobile device IDs, in theory, allow mobile marketers to map the full user journey.
The age of opt-in attribution
In June 2020, Apple announced the end of “opt-out” attribution at the user level and introduced a new form of attribution called SKAdNetwork for all iOS users who didn’t want their device behavior tracked for marketing purposes. Before June 2020, iOS users could enable Limit Ad Tracking to opt out of tracking. Come April 2021, Apple rolled out in iOS 14.5 App Tracking Transparency (ATT), a mandatory in-app prompt that requires every app to explicitly ask users for their permission to be tracked or not.
Suddenly, mobile marketers no longer had access to the majority of users’ IDFA data, throwing the entire mobile marketing industry into chaos.
Mobile attribution today
Today, SKAdNetwork is the de facto attribution solution on iOS.
Since ATT’s release in April 2021, iOS users have overwhelmingly chosen to not be tracked by apps. According to numbers from MMP provider Branch, only 5.38% of all installs tracked through their platform were attributed via MMP attribution compared to the pre-ATT baseline. This means that the vast majority of iOS users are being attributed via SKAdNetwork, Apple’s attribution solution. SKAdNetwork is a last-touch, deterministic, campaign-level attribution solution. The deterministic aspect of SKAdNetwork is key here and we’ll discuss why later.
Chart 1: Successful attribution rate tracked by Branch. Chart compares the percentage of successfully attributed installs compared to a pre-ATT baseline. This gives the best measure of ATT’s impact on MMP attribution rates.
On Android, MMP attribution still reigns supreme. Although we’re increasingly seeing platforms such as Facebook and TikTok limit the level of reporting that MMPs can offer to their customers, restricting reporting of user-level attribution through policy decisions similar to Apple and their SKAdNetwork constraints. Mobile marketers can expect to see Google follow Apple in significantly limiting device ID access from Android users over the next couple of years and see Google Ads follow Facebook by limiting the user-level reporting granularity that MMPs can share.
Alternative attribution methodologies
Multi-touch attribution
Multi-touch attribution (MTA) is the holy grail of attribution.
If the goal of attribution is to “give the right amount of credit to a campaign or channel/ad network for driving downstream marketing metrics such as site visits, installs, or purchases,” then MTA aims to accomplish exactly that. Unlike MMP and SKAdNetwork attribution methodologies which are last-touch models, MTA credits every touch.
From the user journey example earlier of a user who “viewed an ad for an app on Facebook mobile, but then searched for the product on Google mobile search and clicked there to install the app”:
- Last-touch attribution means the campaign or channel (Google) that drove the final click or view before the user installed the app gets 100% credit for converting the user
- An MTA attribution model attempts to give credit to Facebook and Google based on the influence each campaign had on the user downloading the app
MTA is every data scientist’s dream.
But it’s exactly that—a dream.
The continued assault from mobile platform holders—Apple and Google—and ad networks on user-level attribution have dashed all hopes for the dream of MTA becoming a viable attribution solution to become reality.
Without understanding the full user-level journey across campaigns and ad networks at the device ID level, there’s no way to properly model MTA.
Media mix models versus deterministic attribution
Let’s take a step back and unpack why attribution is important. Attribution helps marketers understand the effectiveness of their marketing investments.
By understanding the effectiveness of marketing investments, mobile marketers can:
- Optimize campaigns
- Assess the performance of different target audiences
- Assess the performance of creative
- And most importantly, allocate budgets effectively at the ad network level
As explained earlier, SKAdNetwork and MMP are both deterministic attribution methodologies. Knowing with 100% certainty which campaign drove the last touch is critical to understanding the comparative performance of platforms, ad networks, and campaigns. This data gives marketers a “bottoms-up” foundational measurement insight into which campaigns were responsible for driving installs and which drove early revenue. Marketers can use this deterministic data to model long-term returns from both SKAdNetwork- and MMP-attributed campaigns.
The alternative to deterministic attribution methodologies is what we’d call probabilistic attribution or media mix model measurement. A media mix model is used to model the effectiveness of campaigns and ad networks without taking into account the underlying deterministic attribution. Media mix models apply a “tops-down” approach to measuring marketing effectiveness and were popularized by offline marketers who couldn’t attribute their marketing investments down to purchases.
A media mix model for mobile marketing purposes takes top-of-the-funnel events, such as impressions and clicks from campaigns and ad networks, and uses this data to attempt to model the downstream impact on installs, in-app engagements, and revenue. With this model, a marketer would aim to attribute the downstream events to ad networks and campaigns, while constantly running tests to determine the accuracy of the model.
The challenge with this approach is that there’s a considerable amount of noise in bottom-of-the-funnel events, where accurately tying impressions and clicks to installs and revenue becomes a challenge. Lift tests quickly become costly once marketers consider the number of channels and the variation in spend required to get statistically significant results for each.
Deterministic attribution + media mix modeling = ❤️
Mobile marketers, ignore SKAdNetwork at your peril.
Why?
Because SKAdNetwork offers consistent attribution methodologies to MMP attribution and is, therefore, key to effective mobile marketing investment measurement. Marketers can and should use these attribution models side by side.
Given, however, some of the challenges that exist due to the technical intricacies of SKAdNetwork, it’s important to include more data where available.
Figure 1: A bottoms-up followed by a tops-down approach to marketing measurement and budget allocation for mobile marketing.
Figure 1 (above) shows how AlgoLift by Vungle believes marketers should think about the measurement of their mobile marketing investments. The below outlines the step-by-step approach:
- Collect impressions, clicks, installs, and revenue from ad networks and in-app data
- Model the predicted LTV of MMP and SKAdNetwork campaigns
- Model the tops-down impact of impressions/clicks on downstream metrics
- Model the optimal budget allocation taking into account bottoms-up and tops-down approaches to measurement
- Allocate ad network budgets
- AlgoLift by Vungle’s Intelligent Budget solution then goes back to step 1
With this approach, AlgoLift by Vungle is taking into account all available data from in-app analytics, attribution sources, and ad networks to optimally model ad network effectiveness, and then using this data to improve budget allocation over time.
Install attribution becomes revenue attribution
With the loss of user-level attribution, the goal of attribution is no longer to deterministically tie an install back to a campaign (install attribution). This was always a limited solution to the attribution challenge.
Again, attribution in mobile marketing can be defined as the need to “Give the right amount of credit to a campaign or channel/ad network for driving downstream marketing metrics such as site visits, installs or purchases.” Therefore an attribution model needs to ensure that when given all the available data signals and their relative importance, that the optimal approach is taken to credit downstream revenue to the appropriate campaign or ad network. Only then does a mobile marketer ensure the most effective measurement of marketing investments and optimal budget allocation.